Artificial Intelligence (AI) has come a long way from its conceptual origins, becoming a central pillar of contemporary technological innovation.
The journey of AI began in the mid-20th century, rooted in the quest to create machines capable of thinking and learning like humans. Since then, AI has evolved through various phases of optimism, challenge, and breakthroughs. It’s significantly impacted how we interact, work, and live in the world around us.
Today, AI isn’t just a field of study — it’s an integral part of everyday technology, driving advancements in a wide range of sectors.
What is AI?
AI is the branch of computer science focused on creating machines capable of performing tasks that typically require human intelligence.
In its beginnings, AI aimed to replicate human cognitive functions such as learning, reasoning, and problem-solving within a machine. This foundational goal was rooted in the aspiration to understand and emulate the processes that enable human intelligence.
Today, AI encompasses a broader range of technologies and applications, from algorithms that can:
- Analyze large datasets for systems that understand and generate human language
- Make decisions with minimal human intervention
- Recognize patterns and images
The meaning of AI has evolved to include the development of machine learning and deep learning techniques. The goal? Enable computers to learn from data and improve their performance over time without being explicitly programmed for each task.
Early Concepts
The origins of AI trace back to ancient myths and stories where humans first dreamt of creating intelligent machines.
This fascination with mimicking human intelligence evolved over centuries, finding a place in scientific discussions and literary works that imagined sentient machines as both helpers and cautionary figures.
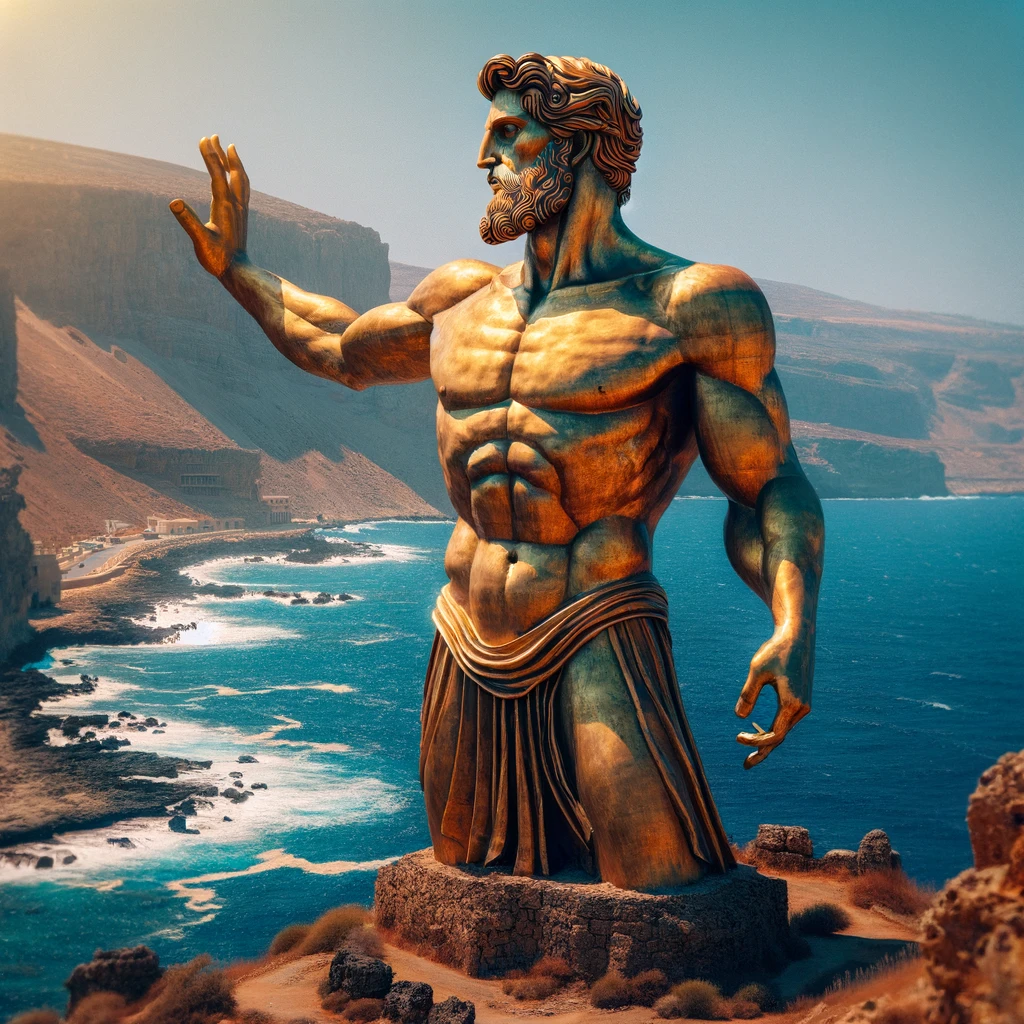
However, dreamwork and imagination started to become a reality as humankind approached the mid-20th century.
How It Began: 1950–1965
In 1950, Alan Turing published his seminal paper “Computing Machinery and Intelligence,” introducing the Turing test as a criterion for machine intelligence, which sparked widespread interest and debate on the potential of machines to exhibit human-like intelligence.
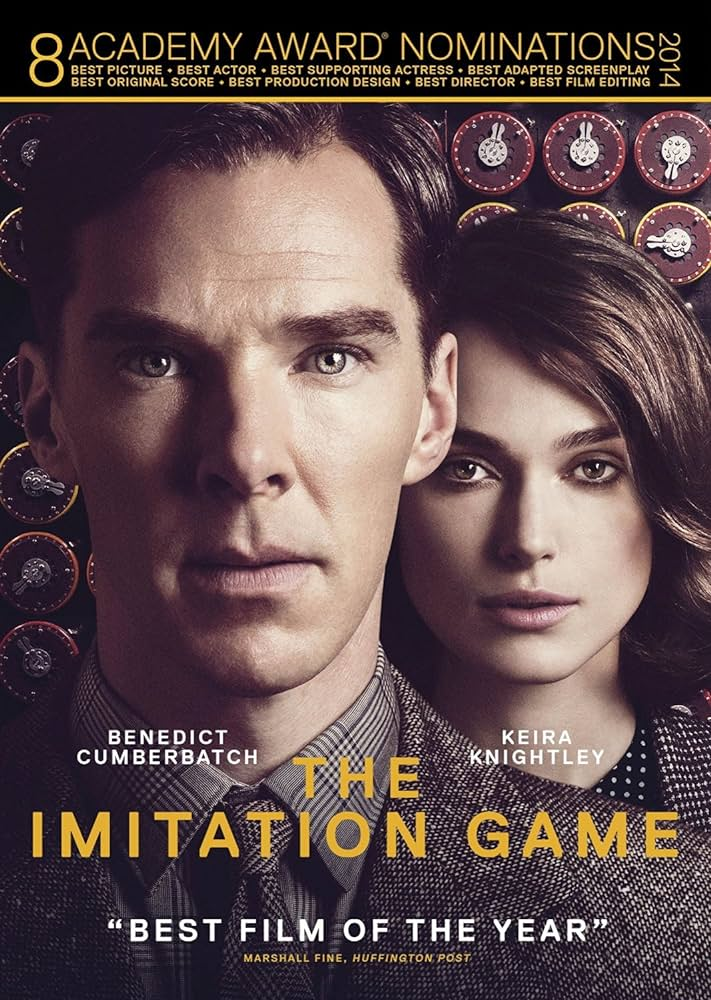
This period also saw the creation of the first computer programs that could play checkers and chess, hinting at the potential for machines to make decisions and solve problems.
Claude Shannon and Norbert Wiener, among others, made significant contributions to information theory and cybernetics. This development laid the theoretical groundwork for AI by exploring how machines could mimic human decision-making processes.
The most pivotal event came in 1956, with the Dartmouth Conference, where a group of scientists coined the term “artificial intelligence.” They proposed that “every aspect of learning or any other feature of intelligence can in principle be so precisely described that a machine can be made to simulate it.”
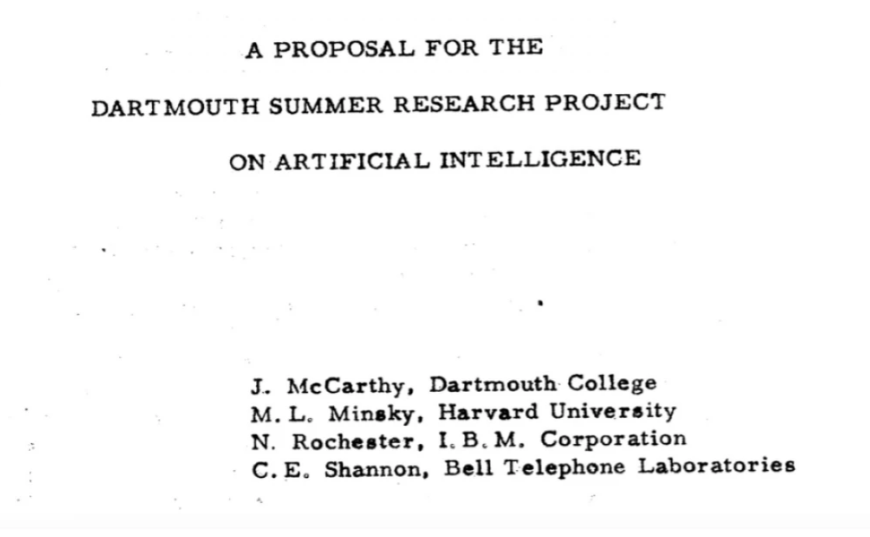
This conference is widely considered the official birth of AI as a field of study, bringing together key figures who’d drive AI research forward.
Cybernetics
Between 1957 and 1980, the development of AI entered a period of significant growth and diversification, marked by the creation and adoption of terms like "machine learning" and "expert systems."
Machine learning has emerged as a subfield of AI. It focused on developing algorithms and statistical models that enabled computers to perform specific tasks without explicit instruction. Instead, it relies on patterns and inferences derived from data.
Concurrently, expert systems were developed, referring to AI programs that simulate the decision-making abilities of a human expert in a specific field.
These systems relied on rule-based knowledge and inference engines to solve complex problems in areas such as medical diagnosis, geology, and engineering.
The period was also characterized by significant advances in hardware and computational power, which facilitated the development and deployment of these complex AI systems.
Institutions like Stanford University, MIT, and Carnegie Mellon University have become key centers for AI research, attracting government and industry support.
This era laid the groundwork for the application of AI in real-world problems, demonstrating the potential of AI to transform various industries through machine learning and expert systems.
The Boom and the Winter
The period from 1981 to 1987, known as the "AI Boom," was characterized by a surge in optimism, investment, and development in AI.
During these years, governments, particularly in the United States, Japan, and European countries, significantly increased their funding for AI research. Why? They were motivated by the potential of AI to revolutionize technology.
This era saw rapid advancements in expert systems and machine learning, with AI applications beginning to show practical value in:
- Medical diagnosis
- Manufacturing
- Finance
The increased value led to heightened expectations and commercial interest.
However, the boom was relatively short-lived. It was followed by a period known as the “AI Winter” from 1987 to 1993, marked by reduced funding, disillusionment, and skepticism towards AI.
The high expectations set during the boom were unmet, leading to a significant decrease in investment from both the government and private sectors. Many AI projects didn’t deliver on their promises, primarily due to:
- Lack of understanding of AI’s complexities
- Technological limitations
- Computational power
Intelligence Agents, AI, and Large Language Models
Between 1993 and 2011, AI and machine learning experienced a period of steady progress and renewed interest.
It eventually moved beyond the challenges of the “AI winter” period thanks to advancements in algorithms and computational power. To reiterate, these were both bottlenecks during the AI winter.
Developing and refining machine learning techniques, such as support vector machines, neural networks, and reinforcement learning, was crucial in advancing AI capabilities.
Also, the internet’s rapid expansion provided unprecedented access to vast amounts of data. As a result, it fueled the growth of big data analytics and enabled more sophisticated and accurate machine learning models.
AI began to make its mark in various fields, from financial services with fraud detection systems to retail with personalized recommendations.
A major milestone was IBM’s Deep Blue, the first computer system to defeat a reigning world chess champion, Garry Kasparov.
This achievement demonstrated the sophisticated strategic thinking capabilities that AI systems could achieve. Deep Blue’s victory was made possible through advanced hardware capable of evaluating 200 million positions per second. Plus, the AI algorithm could anticipate and counter the complex strategies of a grandmaster.
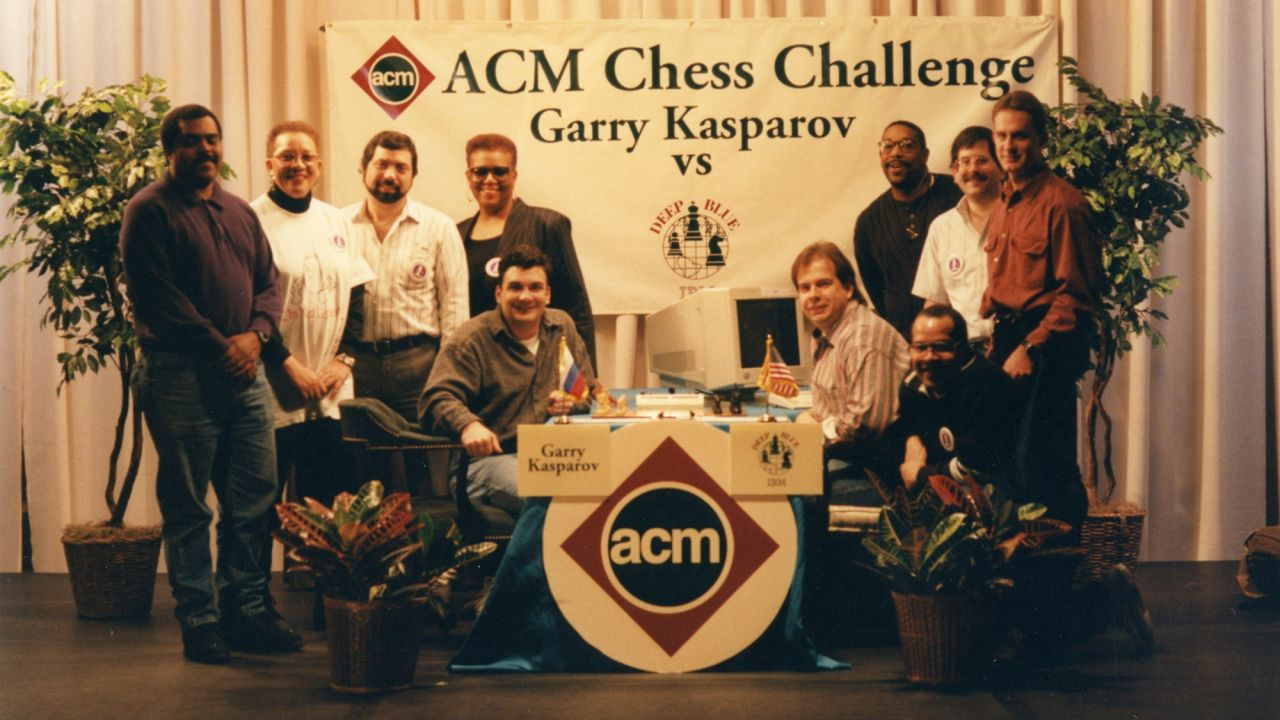
The late 1990s and early 2000s also saw the rise of natural language processing (NLP) applications. NLP technology allows machines to:
- Read text
- Hear speech
- Interpret it
- Measure sentiment
- Determine which parts are important
- Respond in a human-like manner
AI Today and Tomorrow
From the early 21st century to today, AI and machine learning have undergone a transformative expansion, marked by breakthroughs that have propelled these technologies from niche research interests to ubiquitous components of daily life and industry.
This era is distinguished by the ascendancy of deep learning, a subset of machine learning characterized by algorithms known as neural networks, which mimic the structure and function of the human brain.
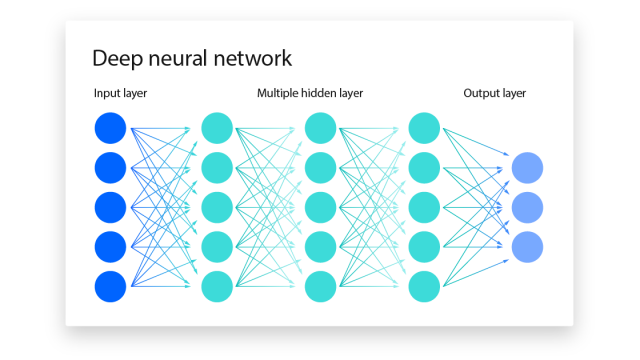
These networks have grown increasingly complex and capable, enabling major advancements in computer vision, NLP, and autonomous systems.
In industries like healthcare, AI is now used for predictive analytics, personalized medicine, and medical imaging analysis, vastly improving diagnostics and treatment plans.
The financial sector leverages AI for fraud detection, risk management, and customer service, optimizing operations and safeguarding assets. For instance, many banks now offer robo-advisors. They use AI and complex algorithms to invest consumers’ money according to individual consumers’ goals and risk tolerance.
Additionally, in marketing, AI generates text, images, and graphics. Among these tools are AI translators or chatbots, facilitating seamless communication across languages.
Overall, AI has become part and parcel of daily business life, being used by 35% of businesses, with 9/10 supporting AI in its ability to give them a competitive advantage.
Potential AI Threats
AI threats aren’t as dramatic as depicted in the movies — but they can be just as devastating.
Deepfake AIs pose significant threats to personal privacy and security. Deepfake technology uses sophisticated AI algorithms to create highly realistic video and audio recordings.
In other words, it makes it easy to manipulate content to convincingly replace one person’s likeness or voice with another.
This capability has been misused for creating false narratives, non-consensual pornography, and fake news, undermining individuals’ privacy and contributing to misinformation.
The potential harm of such technologies highlights the importance of adopting protective measures like using a virtual private network (VPN).
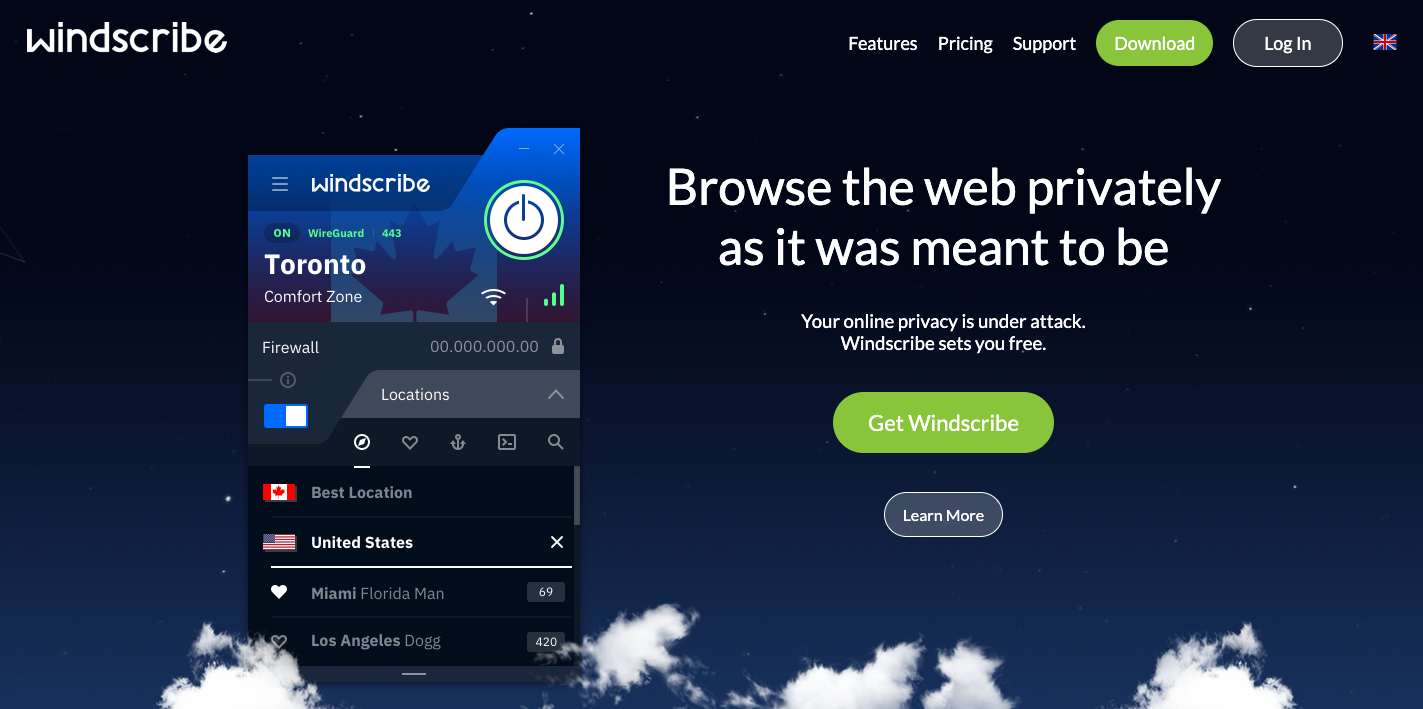
A VPN can help safeguard your online activities from being monitored or traced. It provides an essential layer of security and privacy and can be seamlessly embedded as a browser extension.
Wrapping Up
The evolution of AI from its early conceptual stages to its current integration into everyday technology underscores its remarkable potential and associated risks.
AI has changed industries, made things more efficient, and made big improvements in machine learning and data analysis. However, it has also made it possible for people to use it for bad things like creating deepfake AIs.
The misuse of AI technologies for creating false narratives or violating privacy emphasizes the critical need for protective measures online.
In response to these challenges, employing a VPN like Windscribe is crucial to safeguarding your personal information and online activities. Windscribe VPN provides a secure and encrypted connection, ensuring your online presence is protected from potential surveillance and exploitation.
As AI continues to evolve and integrate into various facets of our lives, it’s imperative to remain vigilant and proactive in protecting our digital footprint.